As we enter the new era of technological innovation, AI is rapidly making its way into our lives. From Open AI’s ChatGPT to Amazon Alexa, we are surrounded by AI today.
But as businesses realize the immense potential and benefits of AI, a new category of Enterprise AI is booming.
According to research, Enterprise Artificial Intelligence (AI) is projected to reach USD 270.06 billion by 2032, growing at a CAGR of 44.1%.
Let’s explore what Enterprise AI is, how it's different from everyday AI and its adoption challenges.
Regular AI vs. Enterprise AI: How is Enterprise AI Different from Regular AI?
Enterprise AI is a subset of Artificial Intelligence designed to operate within large-scale environments. The primary aim of Enterprise AI is to optimize the components of the organizational value chain. The expected outcomes of Enterprise AI include business agility, operational efficiency, and, last but not the least, increased profitability.
Enterprise AI differs from everyday AI because of its specific abilities. This includes integrating with enterprise tools and systems such as Salesforce and Workday. Moreover, an Enterprise AI has to compute large amounts of data each day that a typical AI won’t be able to handle. Some of the most popular examples of Enterprise AI include IBM Watson, Microsoft Azure AI, Amazon Web Services AI, etc.
Now, let us look at Enterprise AI architecture.
How Does an Enterprise AI Architecture Look Like?
Image Source - A sample enterprise AI architecture
Enterprise AI architecture requires careful planning and consideration of different components and technologies, as they all must support business objectives. Here’s a high-level overview of Enterprise AI architecture.
- Data Collection and Ingestion
- Data Storage
- Data Preprocessing
- Model Development
- Model training
- Model evaluation and validation
- Model deployment
- Inference and Prediction:
- Data Governance and Security
- Scalability and High Availability
- Explain ability and Interpretability
- Continuous Monitoring and Feedback Loop
- Integration with Business Processes
- User Interface and Interaction:
- Collaboration and Knowledge Sharing
What Are the Components of Enterprise AI?
1. Data Infrastructure
Data forms the basic foundation of Enterprise AI. There are several kinds of data that organizations deal with, like customer interactions, external data, financial data and operational processes that pile up over time, and hence, it's essential to manage it well. Data warehouses are excellent for managing enterprise data.
2. Natural Language Processing
Natural language processing is a critical component of AI today. NLP helps machines understand human language and interpret it. The most common examples of NLP are Amazon Alexa and chatbots.
3. Robotic Process and Automation (RPA)
RPA uses robots to automate repetitive tasks. This generally includes document verification, form filling, invoice generation, etc. RPA reduces errors and streamlines the processes to boost efficiency.
4. Machine Learning and Deep Learning
Machine learning is a valuable component of AI, as it ensures that your AI system is continuously learning from the data feed and improving its performance. Deep learning also plays a vital role in executing tasks like image processing, speech recognition, etc.
5. Predictive Analytics
Predictive Analytics forms another critical component of Enterprise AI. As companies need to generate valuable insights and forecast trends, outcomes, and events, predictive analytics play a key role here.
6. Cloud Computing
Today, it is no longer feasible to conduct all the operations on premise. Nor is it safe to store data on-premise. That's where cloud computing is helping enterprises. By storing everything in the cloud, enterprises can quickly scale up and scale down their resources.
Industry Challenges with Enterprise AI Solutions
Despite the advantages of adopting AI, several challenges hinder the adoption process. Let’s look at these challenges in detail.
1. Algorithmic Bias
Algorithms are made from data that they are fed. Hence, when a large amount of data from a similar group is used, it can generate a bias toward it, and this can be detrimental, especially in areas like traffic systems and healthcare.
One way to tackle this issue is by creating an ensemble model by combining algorithms trained on smaller datasets and using them as training data for the larger ensemble model.
2. Data Security
Since data forms the foundation of the algorithm, it's critical to keep data safe from manipulation and theft. One of the ways to solve this issue is by using enterprise cloud solutions or blockchains as they encrypt the data. Also, the algorithms must be designed to protect themselves. For example, anonymizing health records.
3. Lack of skilled workers and higher development cost
Developing Enterprise AI solutions is costly. Training an algorithm for ChatGPT costs upward of 1.4 million USD. And the lack of skilled workers adds to the fuel. You will need to invest in workers so they can learn technologies like machine learning and deep learning. The solution to this issue is to partner up with reliable companies that have access to talented workers. You can also partner up with universities that are working towards AI learning.
Principles To Follow While Building an Enterprise AI Solution
#1. Unify Data
As an enterprise, you usually deal with varied kinds of data, including customer data, transactional data, machine data, master data, etc. Since AI solutions require structured data, you must unify data from multiple sources to gain the desired output.
#2. Ensure Data Governance and Security
There’s always fear when it comes to AI. Hence, Enterprise AI platforms must provide robust encryption, authentication, and authorization controls. Everything, including data objects, methods, and algorithms, must be subject to authorization for extra safety.
#3. Leverage Microservices Throughout the Organization
A well-structured AI-based microservice allows developers to effectively build applications governed by security and enterprise protocol. Microservices also aid in scalability.
#4. Promote Team-based Application Development
Usually, the workload of data management falls on the shoulders of data analysts and scientists. An ideal Enterprise AI system aids in solving this issue and allows everyone to work on the application development, thus resulting in rapid deployment.
Top Use Cases of Enterprise AI
1. Airbnb leverages AWS to manage cloud pricing
Airbnb is one company that uses AI to optimize pricing on AWS. Moreover, it uses AI to manage capacity, build custom costs, usage data tools, and optimize storage and computing capacity.
2. AI in predictive maintenance
General Electric(GE) regularly uses AI for predictive maintenance. It analyzes data directly from aircraft engines to identify problems and the needed maintenance.
3. Financial Accounting and Reporting
Intuit uses AI for data analysis to help clients with financial planning. It processes around 730 million customer interactions per year and 58 billion machine learning predictions per day using its Generation AI operative system. OS allows them to implement massive financial models that are customized for tax, accounting, and cash flow.
Conclusion,
Enterprise AI is rapidly making inroads within organizations and changing how things have worked in the past decade. After all, the benefits are countless - streamlined operation, enhanced ability to identify trends, improved products and services, and so much more!
But throughout the transition, you will require support of enterprise AI experts and hire AI developers who have hands-on knowledge, so you can move in the right direction. At Clarion Technologies, we support every step of the AI implementation, so you can recognize its full potential to scale smarter.
Author
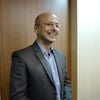